High-Performance Vector Search at Scale
Powering the next generation of AI applications with advanced, open-source vector similarity search technology.
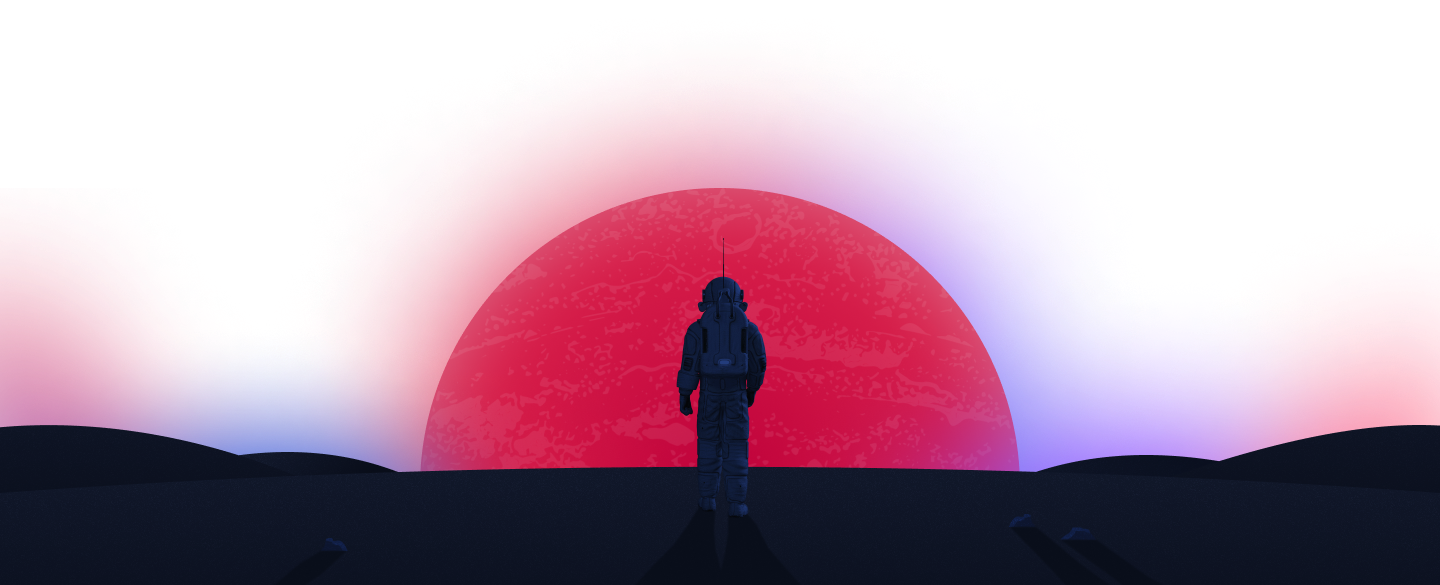
AI Meets Advanced Vector Search
The leading open source vector database and similarity search engine designed to handle high-dimensional vectors for performance and massive-scale AI applications.
Cloud-Native Scalability & High-Availability
Enterprise-grade Managed Cloud. Vertical and horizontal scaling and zero-downtime upgrades.
Qdrant CloudEase of Use & Simple Deployment
Quick deployment in any environment with Docker and a lean API for easy integration, ideal for local testing.
Cost Efficiency with Storage Options
Dramatically reduce memory usage with built-in compression options and offload data to disk.
Rust-Powered Reliability & Performance
Purpose built in Rust for unmatched speed and reliability even when processing billions of vectors.
Deploy Qdrant locally with Docker
Get started with our Quick Start Guide, or our main GitHub repository.
1 docker pull qdrant/qdrant
2 docker run -p 6333:6333 qdrant/qdrant
Vectors in Action
Turn embeddings or neural network encoders into full-fledged applications for matching, searching, recommending, and more.
Advanced Search
Elevate your apps with advanced search capabilities. Qdrant excels in processing high-dimensional data, enabling nuanced similarity searches, and understanding semantics in depth. Qdrant also handles multimodal data with fast and accurate search algorithms.
Learn MoreRecommendation Systems
Create highly responsive and personalized recommendation systems with tailored suggestions. Qdrant’s Recommendation API offers great flexibility, featuring options such as best score recommendation strategy. This enables new scenarios of using multiple vectors in a single query to impact result relevancy.
Learn MoreRetrieval Augmented Generation (RAG)
Enhance the quality of AI-generated content. Leverage Qdrant's efficient nearest neighbor search and payload filtering features for retrieval-augmented generation. You can then quickly access relevant vectors and integrate a vast array of data points.
Learn MoreData Analysis and Anomaly Detection
Transform your approach to Data Analysis and Anomaly Detection. Leverage vectors to quickly identify patterns and outliers in complex datasets. This ensures robust and real-time anomaly detection for critical applications.
Learn MoreGet started for free
Turn embeddings or neural network encoders into full-fledged applications for matching, searching, recommending, and more.
Start Free